The 2012 BPIC Challenge – Process-Mining Driven Optimization of a Consumer Loan Approvals Process
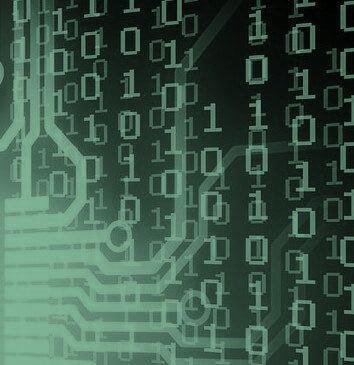
5 min read
Introduction
The 2012 Business Processing Intelligence Challenge (BPIC 2012) was an exercise in analyzing a set of real-world data from a financial institution in the Netherlands. This data set, comprised of 262,200 events within 13,087 total cases, contained information for a loan and overdraft approvals process from submission to eventual resolution (Approval, Cancellation or Rejection).
Using a combination of dedicated process mining tools and traditional spreadsheet-based analysis, we identified a number of opportunities for improvement and key areas for investigation for further process optimization.
Baseline for the Current Approvals Process
In its raw form, the event log is a complicated, yet rich source of process information:
Number of Cases:13,087
Number of Events: 262,200
Distinct Activities:36
Distinct Paths: 4,366
Average Events per Case: 20.03
Average Case Duraction (Days): 8.62
Case Duration (Range, Days):0 – 137
Sorting the cases by eventual outcome (Figures 1 and 2) provides a comprehensive view of the application process, and marks how each of the 13,087 cases are disposed at each of the process steps. From these analyses we observe several baseline performance characteristics:
- About a quarter of applications (3,429 of 13,087) are instantly declined, indicating tight screening criteria for moving an application beyond the starting point
- Nearly a quarter of the remaining applications (2,290 of 9,658) are declined after initial follow-up, indicating a continuous risk selection process at play
- About 23% of applications proceeding to the validation phase (754 of 3,254) are declined, indicating possibilities for tightening upfront security at the application or offer stages
Figure 1: Key Process Steps and Application Volume Flow
Opportunities for Improvement
Eliminating Wasted Resources through Optimization of Future Work Effort
Excluding the 3,472 cases that are resolved almost immediately after submission, we sought to identify the point at which additional work effort yields minimal or no return in the form of completed (closed) applications. As depicted in Figure 3, Day 7 appears to represent a critical stage in the process as the minimum number of days required to fulfill all steps from submission to approval. Approvals continue until approximately Day 23, at which point over 80 percent of all cases that are eventually approved have been closed and registered.
At Day 30, there is a significant jump in the number of cancelled applications as those receiving no response from the applicant at the two customer-centric bottleneck stages (completion of preliminary applications and follow-up after transmission of offers) are cancelled as per bank policy. Our analysis revealed that the bank exerts an additional 380+ person-days of effort between Days 23 and 31, only to cancel a majority of pending cases at the end of this period. With additional data about customer profitability or lifetime value and the comparative cost of additional effort, one can determine an optimal point in the process where additional effort on cases that have not reached a critical point contains no further positive value.
Figure 2: End Status-Based Distribution of Applications
Additional Opportunities for Further Investigation
Leveraging Behavioral Data for Work Effort Prioritization
One of the objectives of process mining is to identify opportunities for driving process effectiveness; that is, achieving better business outcomes for the same or less effort in a shorter or equal time period. To this end, we wished to understand whether we could leverage process event data collected on a particular application to better prioritize work efforts, specifically on the fifth day following application submission.
Figure 3: Distribution of Cases by Eventual Outcome and Duration, with Cumulative Work Effort (Excludes 3,472 Instantly Declined Cases)
To investigate this question, we analyzed a total of 5,255 cases that lasted more than 4 days, for which the final disposition was known. We also considered only those events recorded by the bank until the end of Day 4 and used them to calculate the following variables:
- Which stage the application had reached (initial follow-up, completed application, offer)
- How much effort had already been devoted to the application
- How many, and what types of events had already been logged
- Whether the application required an initial follow-up
- Whether the application had been completed and submitted
We used a Classification and Regression Tree (CART) technique to evaluate if we could identify key segments in the test population that were highly likely to be approved / accepted, or highly likely to be cancelled or declined (Figure 4).
One segment, containing 818 cases and represented by Terminal Node 14, contains applications that were initially incomplete and thus unable to be transmitted an offer by the end of Day 4. We found that such “slow-moving” applications had a less than 6% chance of approval, compared to an average of 41.7% for the test population as a whole. A second segment, Terminal Node 1, contains a total of 200 cases that had been handled by 3 or fewer resources, one of them likely a computerized non-human resource. This scarcity of resources may also be symptomatic of “slow-moving” applications, and as such, these cases have virtually zero likelihood of achieving approval.
Feasibly, one could repeat this analysis at various stages in the application life cycle to assist with effort prioritization. This preliminary analysis indicates significant potential to reduce effort on cases that may not reach the desired end state. Further analysis with customer demographics, application details, and additional information on resources who handle such cases will help refine the findings and suggest specific action steps to improve process effectiveness.
Conclusions
The optimization of the loan approvals process highlighted in this challenge is an exercise in streamlining each step of the end-to-end operation. One notable point that creates challenges in building a streamlined process view using automated process mining tools is the amount and complexity of the captured data. If such data is not used with accompanying business judgment, one can get lost in apparent complexity (i.e., 4,366 possible variants for a process that has 6 – 7 key steps). We recommend dealing with such complexities at the time of analysis, using process knowledge and good business judgment, combined with a fair amount of data pre-processing.
Figure 4: Partial View of a CART-Based Segmentation Tree
As part of our analysis, we performed a rudimentary predictive exercise whereby we determined the current status of cases at a point in the approvals process and quantified their chances of approval, cancellation, or rejection. This allowed us to estimate the fate of a case based on its performance and tailor the overall process to minimize stalling at traditional case bottlenecks. While preliminary in its nature, this surely opens the door to more elaborate future modeling exercises, perhaps driven by sophisticated computer programs and algorithms.
The procedures highlighted by the 2012 Business Process Intelligence Challenge elaborate the role and importance of process mining in the modern workplace. Steps that were previously elucidated only after years of practice and painstaking observation can now be examined using a sample set of existing data. As the era of Big Data continues its march toward the business world, we foresee process mining as a central player in the charge toward turning questions into solutions and problems into sustainable profit.
Download additional materials related to this white paper:
Download a PDF version of this white paper summary of our submission to the 2012 BPIC Challenge
Download our full submission to the 2012 BPIC Challenge
About Business Process Intelligence
Business Process Intelligence (BPI) is an area that is quickly gaining interest and importance in industry and research. BPI refers to the application of various measurement and analysis techniques in the area of business process management. In practice, BPI is embodied in tools for managing process execution quality by offering several features such as analysis, prediction, monitoring, control, and optimization.
The Business Process Intelligence Challenge (BPIC) is an event in which participants are charged to extract meaningful knowledge from large and complex event logs. It is organized annually by the IEEE Task Force on Process Mining (http://www.win.tue.nl/ieeetfpm) and draws submissions from all around the world.