Don’t just hope a solution will solve your problem
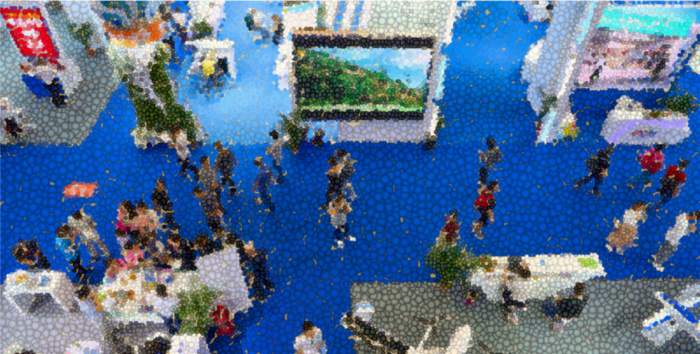
4 min read
Walk into any conference expo, vendor website or sales demo and you’ll quickly find yourself overwhelmed with products proclaiming to provide solutions. In hot spaces like automation and machine-learning/AI the market is abuzz with products claiming to make your operation run better.
Most such products are built on a solid conceptual foundation but fail to translate that into net positive financial impact. Why?
When developing new data science product assets for business operations, we follow a simple sequential framework in positioning assets:
- “You have a problem, and this is where it’s happening”
- “This is why the problem is happening”
- “This is how you fix the problem”
Such concepts are certainly not new to business. Yet despite the simplicity and obvious logic of this thinking many companies frequently fall off-track.
Well-intentioned but misguided leaders often buy products in ‘3’ and skip ‘2’ with the hope or expectation they are fixing ‘1.’ Sometimes they get lucky, but more often they waste millions on ‘solutions’ to problems they didn’t have or that did not matter while failing to make an impact on the things that really mattered. Such leaders forgot to compute the cost of quality and lost a core focus on generating tangible improvements.
The struggles of many companies to return clear value on automation and machine learning investments is a classic case of such missteps. Automation and machine solutions are powerful technologies and work well against specific and well-defined solution spaces—but they’re not magic potions to solve whatever problem you happen to have.
You’ve probably seen something like the following play out:
Company is keen to “keep up with the Joneses” and make sure they’re seen as implementing automation and machine learning, or dare I say ‘AI’ in their operations. Big program announced for an investment in operational automation. Vendors lined up. Slick presentation decks crafted by communicants are used to brief senior stakeholders on the new AI / automation program and all the wonderful things it will bring. A period of time elapses where money is thrown into the program as everyone awaits for all the wonderful things to happen. Fake optimistic metrics are shared but the bottom line does not improve significantly.
Eventually people start to realize that despite millions going out the door the underlying problems broadly still exist. This often conflicts with the metrics and reporting coming out of the program—which cite lots of automation underway across the organization.
Such a company may be quick to highlight the ever-increasing number of ‘automated resolutions’ its operations are realizing. Minor details like the fact that these ‘resolutions’ didn’t actually fix the real problems the program set out to address, or the fact that the automation itself created and then resolved many of its own ‘problems’ are casually overlooked. Ultimately, the company spent millions to create a highly automated mess that hasn’t fundamentally made the operation better, faster or cheaper.
Skipping ‘step 2’ is most often the root cause of the above. Not fully understanding ‘1’ is also often a contributing factor. And, finally, not checking results against the initial objectives is a source of increasing discontent.
So, what should be done differently?
Bringing together the combined digital footprint of data generated by all a business’s operational systems allows for a detailed reconstruction of exactly what is happening when and where. With data science applied to that data, stakeholders can quickly start to understand ‘why’ what is happening is occurring and how that deviates from optimal performance.
Could automation help the operation? Potentially, but what exactly should the automation do? Is the task being automated even necessary? (it would be more efficient to eliminate an unnecessary task than automate it!) What is the true cause of inefficiency in an operation and will the automation even address that cause? What is the projected savings from automation of step X relative to savings from potential investments on other opportunities?
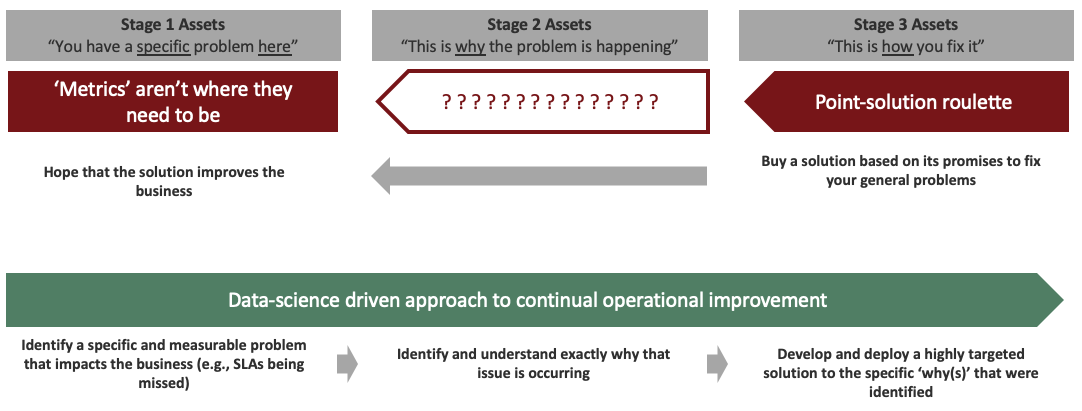
In one simple case study, we recently observed a company investing heavily in automation to fix infrastructure incidents. Millions were spent to deploy automation that automatically flushed log files that filled up, restarted failed batch jobs, and emptied caches. Data science deployed against the combined digital footprint of the operation showed that the real root-causes of these issues were repetitive underlying issues in the environment that were not being addressed by good old-fashioned technology problem management processes.
Rather than changing a line of application code to stop filling up a disk with log files, this company spent millions on automation to automatically empty that disk. A few days later when the same disk filled up again, that expensive automation whipped right back into action to again treat the symptom but not the problem.
Eventually people start to realize that despite millions going out the door the underlying problems still exist.
Metrics from the automation program showed impressive stats on the ‘number of automated resolutions’ and early in the automation program those stats were cited to senior stakeholders as evidence of the program’s success. However, data science quickly revealed these stats to be a thinly veiled cover for a highly unstable infrastructure broadly held together by very expensive automated duct-tape appliers that completely missed the ‘why’ behind the company’s performance struggles.
By following the simple logical ‘1, 2, 3’ framework above data science assets were able to quickly identify that the ‘why’ in this case behind the high volume of incidents distilled down into a relatively small and defined set of issues that could and should be quickly fixed via simple changes to underlying code, processes and practices.
Was there anything fundamentally wrong with the automation products? No. Was it the right solution to the problem the company really had? No. Any tool must integrate 1, 2, 3 because a failed shortcut costs you, not the software salesperson.
In today’s world full of hyped-up solutions looking for problems to solve, don’t forget the fundamentals. While the exact ‘why’ behind your operational struggles may be difficult to see at first, advanced data science approaches can quickly and clearly identify highly actionable insights that can be used to far better match solutions with problems.2
Invest a little in getting the ‘why’ right now and avoid very expensive mistakes later.
Dr. Hartman is the Chief Innovation Officer for CKM Analytix