Asking Questions
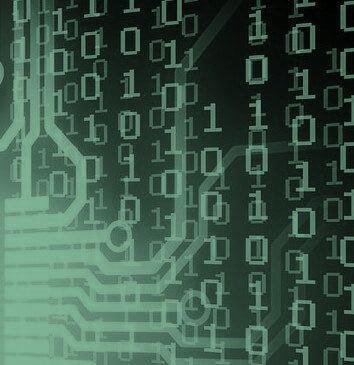
2 min read
Data Scientists today are expected to have a strong grasp of mathematical modelling and computer programming. Increasingly, there is also an expectation that they be able to ground these skills with business acumen. Too often however, we find Data Scientists tend to jump into analyses without taking the time to really think through the problem they’ve been asked to solve. Fortunately, there is a simple antidote to this tendency – asking questions, and with enough practice and experience, asking the right ones.
For us at CKM, this willingness to ask questions, to listen and adjust, is essential. We don’t know all aspects of a new space right away, and working closely with subject matter experts allows us to develop solutions that make the right assumptions and are grounded in operational realities. To take a recent example – we were asked to leverage our data science toolkit to help identify geographic areas at risk of infectious disease outbreaks for a governmental organization. At a minimum, this ask required us to get answers to the following: a) what is the operational definition of risk b) what are the key risk factors c) is there data on these risk factors d) what are appropriate metrics for tracking them, and e) what types of output could drive toward action. Who knew a seemingly simple ask would necessitate so many questions?!
Contrast this approach with a general tendency to jump in and explore readily available data for insight. In the rush to solution, key aspects of the problem get overlooked, ultimately leading to sub-par output that only addresses a portion of the original ask. Instead, what we are advocating is more time spent engaging with the questions, even at the expense of less time analyzing the data. This becomes vital when one is brought in, as we are, to solve often vaguely defined business problems such as improving efficiency or streamlining processes within the context of a large organization; target datasets are large and one can get lost for weeks digging through them to find snippets of insight. To add further complexity, we often must balance findings with broader organizational perspectives and motivations, so as to correctly assess impact and prioritize work. In all cases, not asking these questions will lead to misaligned expectations, time wasted solving for ghost questions, and ultimately an unhappy client.
This willingness to engage fares Data Scientists well in other aspects of their work too. In our experience, individuals who ask the right questions build stronger relationships with their peers, advance rapidly within the organization, and learn more, quicker.
In conclusion, often overlooked humility in the face of new problems, listening and asking questions, are core attributes of a strong Data Scientist.