Big Data on the Big Data Conversation: Tracking the NSA Story
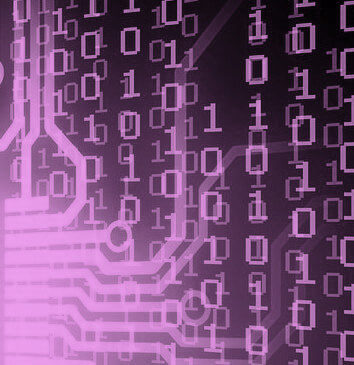
6 min read
Recent revelations regarding the National Security Agency’s (NSA) extensive data interception and monitoring practices (aka PRISM) have brought a branch of “Big Data’s” research into the broader public light. The basic premise of such work is that computer algorithms can study vast quantities of digitized communication interactions to identify potential activities and persons of interest for national security purposes.
A few days ago we wondered what could be found by applying such Big Data monitoring of communications to track the conversational impact of the NSA story on broader discussions about Big Data. This brief technical note highlights some of our most basic findings.
Our communication analytics work is usually directed at process optimization and risk management. However, in this case we applied some of the most basic components of our analytics tools towards public social media conversations – specifically tweets collected via Twitter’s streaming API. Starting last summer, we devoted a small portion of our overall analytical compute resources towards monitoring news and social media sites for evolving trends within various sectors including technology. A subset of this data containing tweets on topics related to Big Data is analyzed below.
Background Data Volume
Twitter’s streaming API provides real-time access to public tweets. The two graphs below show the relative volume of communications collected for conversations related to Big Data (between 30 May – 12 July 2013). The rate of communications collected was highest on weekdays and during working hours (in US timezones). This observation is consistent with the expectation that most of the Big Data conversation takes place in the context of business activities and the companies applying Big Data to business problems. Conversations on other topics (e.g., leisure activities) would have a very different profile.
In the graph below the black line shows the volume of Big Data conversation text monitored between 30 May 2013 and 12 July 2013. Following the overall pattern outlined above, conversations peak in the late morning (EDT) with higher volumes during weekdays. The red line shows the subset of these conversations that discuss key topics related to the NSA story including the NSA itself, the PRISM monitoring program and Edward Snowden. While the NSA story has been making headlines for over a month now, it represents only a tiny subset of the overall day-to-day Big Data conversation that takes place every day across Twitter.
Evolution of the Story’s Breaking Subjects
When Big Data met Big Brother RT @guardian Revealed: NSA collecting phone records of millions of Americans daily http://t.co/YyEV4Tdz1Y
— Stephen Foley (@stephenfoley) June 5, 2013
Big Brother LOVES you. Dear lord.. RT @RosieGray: i am already worried for whoever leaked PRISM http://t.co/3b4AQTsYaH
— Joe Gigante (@joeygigante) June 6, 2013
Prior to 5 June 2013 the NSA was only occasionally mentioned in the context of conversations discussing Big Data. Such references typically included publicly discussed analytical advances or a widely reported new data center in Utah.
Starting at 7:19 PM on the evening of 5 June 2013 our language analysis algorithm picked up a sharp increase in a new conversational topic related to the NSA. The first tweet detected by our language algorithm is shown at right. As you may recall, the first tidbits about the current NSA story came from the Guardian’s report of phone records being recorded.
This conversation traffic remained relatively constant for the next 24 hours and then exploded in volume the following evening starting a 6:08 PM, when the second wave of the NSA story broke via the leaking of PRISM.
The graph below shows the evolution of communication volume about these topics as they related to conversations on Big Data. The NSA conversations (in red) are immediately joined by the conversations about PRISM (in blue) once the second wave of the story broke on the evening of June 6th. Conversations on PRISM reduced considerably in the late evening and then resumed in full force again the following morning as the AM news cycle kept the conversation going. While the news of PRISM took the Twittersphere by storm, it apparently didn’t keep people up that night (or at least if they were up, they weren’t tweeting).
As you’ll recall, the source of the leaks was not publicly revealed until several days after the NSA story broke. Edward Snowden’s name is first picked up by our language analysis algorithm on the afternoon of 9 June 2013, which is when he came forward as the source of the leaks. Big Data conversation traffic related to Snowden (in green) remained minimal relative to conversations on the NSA and PRISM. While Snowden’s legal troubles were and remain big news, our language analysis indicates that conversations on those issues remains largely separate from broader conversations on the data and technology aspects of the story.
Embroilment of Technology Companies in the Story
Many technology companies have been directly and indirectly associated with various aspects of NSA story. Some of these initial associations were spurred by leaked documents published in the news media, while other associations mentioned in conversation are the result of pure speculation. Of these companies, some (e.g., Microsoft) are frequently mentioned on a daily basis as part of the overall conversation about Big Data technologies.
During the evolution of the NSA story, we wondered how much of the public conversation related to these companies and their work in advanced data analytics was related to the NSA story. In other words, how ’embroiled’ were each of these companies in the story (within the context of Big Data and data analytics).
To study this question we first looked at the overall volume of conversation related to advanced data analytics and different companies. The chart below shows the relative volume of such observed communications relative to the volume observed for IBM (a firm frequently mentioned in the context of advanced analytics). The scope of companies shown is certainly not exhaustive, but represents a broad spectrum of technology firms. Not surprisingly, Google stands out in this list as another dominant company mentioned in the context of advanced data analytics.
We next calculated a basic “embroilment factor” for each of these companies. This simply measures the percentage of conversations discussing the company and data analytics that do so in the context of the NSA story. The chart below shows this measurement on a weekly basis starting with the week before the NSA story broke and continuing for 5 weeks afterwards. Although IBM was mentioned far more than any of the other selected companies in overall conversations about advanced analytics, it was barely mentioned at all in relation to the NSA story.
In contrast, Microsoft was initially mentioned in the leaked PRISM documents causing an association with the NSA story that lasted for about 2 weeks. Even during the peak of the NSA story’s news cycle about 90% of observed conversations about Microsoft and advanced analytics were not discussing aspects of the story. The initial embroilment association had mostly disappeared from conversational language after about 4 weeks, but then earlier this week an additional story broke that again mentioned Microsoft in relation to the NSA story. This caused a resurgence in the embroilment factor over the last few days.
Visualizing the Conversation
Finally, we visualized the time evolution of conversational discussion topics. The simplified network animation below shows the relative volume of Big Data conversations mentioning the NSA story and the selected group of technology companies (both with and without association to the NSA story). Within the NSA story itself, the conversational associations of the three major subjects (NSA, PRISM and Snowden) are shown. Commentary on a few selected frames in the animation are also included below.
After the main PRISM story broke (see Figure 1) there was a clear conversational linkage between the NSA and the PRISM technology identified by the press. The leaked classified documents also referenced a range of technology companies that then also quickly became associated with the NSA story (leading to a high ’embroilment factor’ as discussed above). Many of these associations were the result of leaked slides in a NSA presentation, while others were the result of pure speculation on behalf of the news media and/or individuals. At this point in the timeline, Edward Snowden’s identity was not yet revealed and thus not surprisingly an analysis of his name on this date reveals no conversational association with the NSA or PRISM (note: the heavy red line at the bottom is indicating traffic to the overall NSA story, not Snowden).
A few days later (see Figure 2), Snowden’s identity was published by the media. This immediately changed the direction of the story and resulting conversations towards Snowden. Not surprisingly, language analysis now shows an extremely strong association between Snowden and the NSA/PRISM. The volume of conversation including most technology companies has also dropped off rapidly relative to the previous few days.
Conclusion
In this technical briefing we outlined the results of some basic language analysis on conversations related to the ongoing NSA/PRISM story. This simple overview just scratches the surface on the power of advanced analytics for studying the ‘digital footprint’ of a topic. When responsibly and ethically applied in a business environment, such technologies can provide a paradigm shift in the ability to efficiently complete and manage complex processes. For example, language analysis of log entries on IT incident tickets can (when coupled with process mining) provide extensive insights into nature of key challenges facing a technology organization.