Data Isn’t Just Data
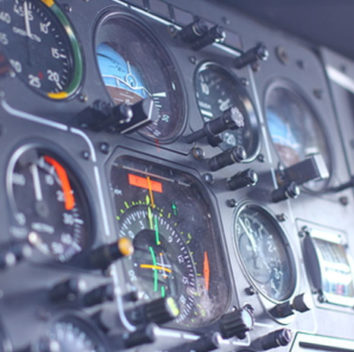
4 min read
“We are data driven.” “Let’s look at the data and focus this conversation on the facts.”
There’s no question that people are paying a lot more attention to their data today. Determining the leaders and laggards in an industry increasingly boils down to who is best at translating their business data into business value.
While I’m undoubtedly a strong proponent of looking at the data, CKM’s extensive experience studying data across different operational processes along with formal training as a scientist and a pilot have taught me that things aren’t just all about the data.
How is a data point really created?
Scientists are trained to study and interpret data but also to be highly skeptical of where it comes from, how it was generated, and what it really means. Consider a simple data point like temperature, which is collected from an instrument like a thermometer. While temperature seems like a simple concept, determining the temperature only takes place indirectly via measurement of other physical properties—such as the volume expansion of liquid mercury or the electrical resistance of a material.
Take the electronic version. A temperature change alters the electrical resistance of some material, but that too isn’t something that just results in a data point. We need to conduct an additional experiment to measure what the resistance of that material is, which means we need to supply a known voltage (which needs to be created) and measure the current passing through a circuit. We compare that reading against some model of how resistance varies according to temperature. We then need to transmit that measurement somewhere to either be recorded or displayed. Every one of those components and processes could have some potential error, and of course the whole system has some lag associated with it too—it may take a few minutes until the readings are accurate after a rapid temperature change.
Scientists scrutinize their equipment for such factors to understand how measurements are made and the potential for error in such measurements. Very good analytical scientists typically take the attitude that every component is broken and conduct testing to demonstrate that it’s not before proceeding with an important data collection. Within business such rigor is often ignored, and data points are taken at face value as being correct.
Take a timestamp in a ticket citing the time a technology service ticket was “resolved.” What is that really saying? Is it absolutely the time the ticket was “resolved?” Probably not. It’s probably the time when a person clicked a button saying the issue was addressed. Did that click happen seconds after the fix took place? Maybe 45 minutes later when a technician returned from lunch and sat down to close out some old tickets? Maybe the issue isn’t fixed but someone is clicking “resolved” to fiddle with the data used to calculate SLAs so next week’s management update PowerPoint deck says an SLA was met? (I’ve seen that one a few times!)
Data science teams should always think about every bit of data as having come from an instrument. It’s not a “resolved timestamp instrument” but rather an instrument that records when an agent pushes the resolved button in ServiceNow. Is that an accurate proxy measurement of the true resolution time? Maybe, maybe not.
The data often exists to dig deeper and study what that time really means, but few make the effort to challenge the data and dig deeper. These best uses of operational data always scrutinize any such data points and leverage all available other data to cross-check information streaming out of any one source—which is my next observation.
Keep up a good scan of your instruments
Instrument rated pilots are trained to fly and navigate an aircraft with no visible reference to the outside world (flying inside the clouds). Here, the lives of those onboard the aircraft depends on the ability of the pilot to interpret data from onboard instruments to get from A to B and keep the plane right side up—which thanks to the quirks of our inner ear is a surprisingly difficult thing to do without data from instruments.
However, just like the scientific instruments discussed above, aircraft instruments can also fail or give inaccurate readings. Fixating on one instrument and its data can be very dangerous—especially if that instrument is outputting bad data. Pilots are trained to keep up a consistent scan of all the instruments, and their data, and mentally cross-check streams of data for something that doesn’t make sense—for example, altitude pitched down and airspeed increasing (indicating a decent) but the altimeter indicating level flight. Pilots train to quickly identify such scenarios, isolate the problematic instrument/data and develop an alternative plan to determine required data (like altitude) and get the aircraft and its passengers safely back on the ground.
In business, this concept is too often skipped. Reports are generated from data and action taken from those reports often without cross-checking or sense-checking the data. Such stakeholders get fixated on one ‘instrument’ and don’t keep up a broader scan. Taking this approach in the aviation scenario above—blindly following the bad altimeter reading—is a great way to fly into the side of a mountain in fog. The business equivalent of an incomplete instrument scan can quickly get a company into trouble.
One of my favorite scenes from the film Apollo 13 is when, shortly after the spacecraft experiences its explosion, the engineers back in mission control are perplexed by the data streaming back in. One engineer fixated on his screen says “It’s reading a quadruple failure. That can’t happen. It’s got to be instrumentation!” but the flight director is looking at the bigger picture and quickly tells the room “These guys are talking about bangs and shimmies up there, doesn’t sound like instrumentation to me!” Leveraging advanced data science doesn’t mean we should stop paying attention to what people are hearing and saying—that’s data too! When every instrument seems to conflict with each other sometimes just asking people what’s happening is the best data you have in that moment.
Applying these lessons
Understanding where data comes from and how it’s generated is key to any use of data—especially up front when new systems and analytical approaches are designed. Take data at face value at your peril. Maintaining a good scan and a broader situational awareness is key, especially when interpreting the results of analysis. If someone markets a ticket as ‘resolved’ but log files show the technician is still tinkering with the impacted asset, a good scan will pick that up and know to challenge the ‘resolved’ data point.
Maintaining situational awareness and understanding where data comes from should be central to the collection and use of data within your business. These lessons should be incorporated into all the tools, processes and advanced analytics that leverage that data. Both are also continuous mindsets vs. one-off audits or something only done up front. Applying that continuous approach creates a strongly positive re-enforcement loop that drives continually-improved instrumentation and better analyses of the data coming out of those instruments.