Check Your Models, ‘Normal’ Has Changed
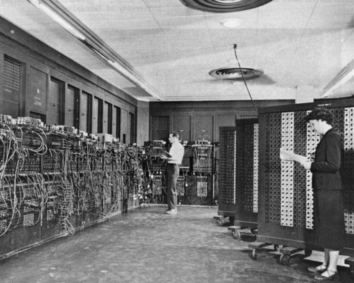
4 min read
This morning I received an e-mail from my gas and electric company. I won’t name names but it’s one of the big ones. The subject of the e-mail said “Unusual energy usage detected” and the message outlined that artificial intelligence monitoring the energy usage from my ‘smart meter’ noticed my electricity usage had recently increased by 32% above ‘normal.’
The e-mail goes onto describe analysis outlining possible reasons for this ‘abnormal use.’ It said “The weather has been similar to this time last year, and may not have affected your bill” and goes on to describe that “Factors like heavy appliance use or household guests may have contributed.” It then went on to describe some things I could do to save energy, like opening the curtains and letting more natural light in to avoid turning on the lights.
Of course the reason why my household is using 32% more electricity than last year is because our whole family is currently home nearly 24/7, including working from home. Our home office, normally cold and dark during the day, is now a hive of activity with two adults conducting business all day long and a child nursery operation on the side to take care of our little one.
The e-mail was an amusing distraction but highlights a core point in deployment of machine learning and so-called artificial intelligence solutions into business operations. For one, such technologies have a difficult time understanding that ‘normal is no longer normal.’ The global COVID-19 situations has completely upended nearly all aspects of our society, business and personal lives.
Supply and demand models? Need to be complete re-worked. Creditworthiness models? Probably very wrong now. Models predicting traffic movements? The roads are mostly empty.
Even fairly sophisticated mathematical models can easily miss that their detected ‘anomaly’ is in fact simply a new normal, or fail to predict anomalies that are clearly coming down the pipeline.
A few years back I had traveled to South Carolina to watch the total solar eclipse. I had budgeted plenty of time to drive back to Charlotte to catch my flight back to New York and when I got in the rental car a very popular navigation app on my phone assured me that it’s prediction of traffic levels showed me arriving in plenty of time to relax and catch dinner at the airport before my flight. As the drive progressed the predictions slowly got worse and worse and before long I was at a near standstill on the highway. After originally telling me I would arrive nearly 3 hours before my flight it said I would arrive 10 minutes after my flight was due to depart. Oops.
Turns out the ‘traffic model’ clearly wasn’t considering the fact that the unusual pattern of traffic that appeared going into a zone that morning (to watch the eclipse) would reverse later that evening (after the eclipse)–of course I probably should have realized the same and left even earlier! ‘Normal’ traffic patterns on that day were meaningless. There was a very clear and obvious non-normal situation playing out but because the model didn’t consider that broadly known issue it was producing non-nonsensical results. After some adventures across rural roads far off the interstate and a sprint across the terminal I made my flight with seconds to spare.
Of course, the energy model that sent me the e-mail should have noticed that lots of people are showing a sharp increase in energy use and figured out that something deeper than just a change in my household is underway, but hindsight is always 20/20. The real root cause here is the way such models and automated are deployed. Letting any model run fully autonomously is always risky. Sending out silly e-mails is low risk, but imagine if the same model was automatically trying to re-allocate energy across the grid based on ‘normal’ patterns that no longer existed. That could have much more real and tangible negative impacts.
When thinking about deploying models into any operation, regardless of the amount of automation, there are three core things to think about:
- What’s normal?: At some point ‘normal’ stops being ‘normal.’ Do we have safeguards and logic in place to detect that something odd is happening and have the model take itself out of the equation? Sometimes the smartest thing a model can say is “Hey I don’t know what’s going on but someone better take a look at this!”
- Machines are terrible sense checkers: Be very careful of letting models run on auto-pilot without a human in the loop somewhere. General intelligence in AI / machine learning does not exist and likely won’t exist for a very long time. There is simply no replacement for a human’s ability to quickly identify that something “doesn’t smell right.” That level of soft logic and intuition is difficult if not impossible to replicate in code.
- Know when to pull the plug: When there has been a clear shift in ‘normal,’ re-assess the whole situation and decide if the model and its use even makes sense anymore. For example, I would not want to be using a credit or other risk model off the shelf as it were 3 months ago. Normal has changed. Just like assessing what my household’s energy use is, training of the old model largely goes out the window at this point and new thinking is required to understand how such decisions are best made given everything that’s going on in the world today.
Stay safe out there… and check your models!
Dr. Nicholas Hartman is the Chief Innovation Officer at CKM Analytix