Common Statistical Fallacies and How to Avoid Them by Using T-K from CKM
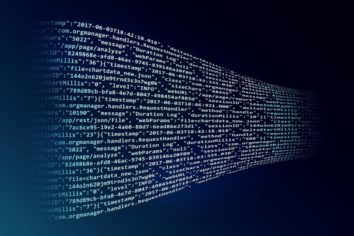
5 min read
The amount of data in the world was estimated to be 44 zettabytes at the start of 2020. If you are wondering, a zettabyte equals approximately a thousand exabytes, a billion terabytes, or a trillion gigabytes – in lay terms, a lot.
Given access to all this data, it is easy to get lost in the numbers. In this article, we’ll be exploring aspects of data literacy that take you beyond just understanding the difference between your means and medians, with a particular focus on the ITSM industry.
Towards this goal, we’ll discuss some well-known data fallacies, point out why they are problematic, and make sure you know how to spot them in the wild and avoid them in your work. We will also show how CKM Analytix’s T-K software product is designed to avoid these common mistakes.
Cherry Picking and The Fallacy of Incomplete Evidence
Cherry-picking involves focusing solely on a portion of data or a specific set of metrics that tell the story you want told while ignoring similar data or other metrics that counter your position. While it can be accidental and is often seen in the context of confirmation bias (searching for and interpreting data in a way that conforms to your existing beliefs), cherry-picking can be a deliberate choice. To avoid being caught up in it remember to:
- Zoom out and look at the bigger picture
- Ensure that no facts or evidence are being portrayed out of context
- Ask questions from a different angle
An example of cherry-picking in the ITSM industry is to look at only certain metrics from your service desk. For example, let’s say that the service desk in question has a really low ASA (average speed of answer). If we leave it there, we may conclude that the service desk is performing well. But what about its FCR (first contact resolution) rate? It may be very low because calls are being cut short to balance incoming demand. Alternatively, the desk may be heavily overstaffed to handle its incoming demand.
The T-K Difference
With CKM Analytix’s T-K software, we always strive to show metrics within the context of a larger picture. Data from multiple sources (ticketing systems, telephony, shifts) is analyzed so that we can avoid drawing conclusions from incomplete evidence.
Bias and Pre-Filtered Data: Fool Me Once…
Survivorship Bias is the logical error of viewing data that has already made it past some selection process as representative of the whole.
The classic example of survivorship bias comes in the form of a story from WWII. The US military wanted to strengthen aircraft for warfare, and to do this they studied the planes that returned from battle. Noting the areas that sustained most of the damage, they proposed to reinforce these areas in particular. Mathematician Abraham Wald looked at the same damage done to the planes and proposed to reinforce the areas that had not been damaged on the returned crafts. The bullet holes, he argued, indicated areas in the crafts which were able to both sustain damage and allow the crafts to return to base. Those planes that were hit elsewhere went down in battle and were unable to return (in other words, became ‘missing data’).
So how can we look at our operational data and take note of what we are not seeing? Let’s think about a service desk logging cases throughout the week. On a Monday morning, they reach a peak of logging 100 cases in an hour, not that different from the rest of the week where they average approximately 80 cases per hour. You would be forgiven for concluding that Monday mornings are not much busier than the rest of the week, but this is not the whole picture. There are a whole set of cases that never make it into the system: cases that would have been the result of abandoned calls – i.e., customers who were waiting too long in the phone queue and hung up before being serviced.
The T-K Difference
This is a great example of survivorship bias that we see when a service desk tries to understand its demand at different points of the week. Demand cannot just be counted from how many cases a desk is able to work on, but also how many cases never make it into their system as a result of customers abandoning calls. CKM’s T-K software reports on holistic demand trends, including data ‘that isn’t there’ – abandoned calls – in order to show a true picture of demand across the day.
Cobras, Observers, and a Paradox: Hidden Data Manipulators
Cobra Effect (Perverse Incentive): named after a historic legend, the Cobra Effect occurs when an incentive for solving a problem creates unintended negative consequences.
Urban myth tells of the British Empire in India trying to find a way to reduce the number of cobra bite deaths. They decided to offer a reward for every cobra skin brought to them, hoping that this would reduce the number of cobras in the wild. Instead, many enterprising individuals started breeding cobras for the reward. When the British heard of this, they canceled the scheme, causing many more farmed cobras to be released into the wild.
Decisions can have unintended consequences, and when a system change is enforced it is important to monitor for any unintended knock-on effects. In a bid to reduce call handle times, for example, a desk’s FCR rate may also decrease as agents spend less time on the phone with each customer. What starts as an efficiency gain could lead to the breaching of a different SLA, which in turn may end up costing more in the long run. Service, efficiency and risk are inextricably intertwined. Gains in one (here efficiency) can be at the cost of another (the customer experience of having issues resolved at first contact), and such changes should not be implemented without considering all the downstream effects.
The T-K Difference
T-K’s Knowledge Graph module links work done on calls and cases between different systems, meaning that you monitor, in real-time, the effect of a decision across your workforce. It also makes suggestions that increase both efficiency and customer experience, such as schedule optimization, helping avoid perverse incentives entirely.
Hawthorne Effect: also known as the Observer Effect, it comes about when the act of monitoring someone can affect that person’s behavior. It was coined from studies at the Hawthorne Works factory outside of Chicago in the 1920s and 1930s. Small changes in lighting were said to bring about increases in worker’s productivity, these levels then slumped again when the study ended. It is now used to refer to any temporary increase in productivity over a short period in which workers are observed.
The T-K Difference
It can be hard to monitor a desk for productivity because agents conduct work across multiple systems, and in the presence of managers are likely to step up their output. But with T-K monitoring across systems without interfering in an agent’s workflow, it is easier to understand agent productivity across desks and isolate agents who are in need of extra training in specific areas.
Simpson’s Paradox: a phenomenon in which a trend appears in different groups of data but disappears or reverses when the groups are combined. It is a particular type of association paradox, and, as is perhaps befitting of this connection, was neither discovered nor claimed to be discovered by statistician Edward Simpson with whom it is associated.
Although a little confusing in definition, Simpson’s paradox is simple enough to understand when looking at an example. If we consider an agent’s productivity overall, we may see that they sit within a lower performance group compared to their peers. However, when we look at productivity broken up by category, we may see that this changes somewhat. This same agent could be particularly effective at dealing with one type of case. Here they far outperform their peers, but as they work on fewer of these cases, this is missed when only the aggregate grouping is considered.
The T-K Difference
T-K provides the option to break up metrics by different categories in order to understand and make use of the knowledge provided by trends across different groupings. This insight from T-K allows an organization to champion an agent’s behavior in some areas while providing training in other specific areas, opportunities that may be missed when performance is only viewed in aggregate.
CKM Reveals the True Character of Your Data
Remember that when working with data, numbers, and figures themselves are not representative of any truth. Ask yourself why you are seeing this set of data – is there anything that you are missing, or that someone has chosen not to show you? Do you have an honest representation of the whole, and understand what has influenced the picture? Is it possible that the figures tell a different story de-aggregated?
Taking time to sense check your data is important, and with a tool like CKM’s T-K you can do so easily and with confidence that you are not falling prey to any data fallacies in the process.