Let’s Talk About ‘Automation’ In Service Operations
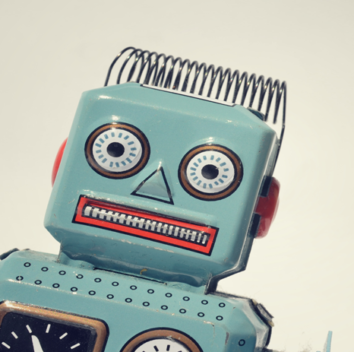
4 min read
Through our work in implementing data solutions around the globe, we have the opportunity to see many large-scale automation programs underway across many aspects of the services sector. We’ve developed an empirically-derived view of what works well and what does not—and we’ve observed some common themes across those successes and challenges.
In talking about service operations, I am referencing any ‘soft’ (i.e. not manufacturing), end-to-end operational process, which can range from call center operations and loan underwriting to HR onboarding.
As we look across any process—and service operations are at their most basic level a collection of processes—we see a consistent hierarchical theme in what works for driving efficiency. Specifically, we use detailed digital footprints derived from operational data to:
A. Eliminate what you don’t need to be doing (those things that return no value)
B. Optimize the remaining process to drive consistency and governance around performance
C. Automate those steps within an optimized process that can be logically and efficiently executed in an algorithmic manner
There are three trends that we’ve consistently observed as large organizations work towards deploying automation across their service operations:
1. You will struggle to effectively automate processes that are not already highly efficient
This is likely one of the biggest issues with automation applied within service operations. Automation is highly unlikely to magically make broken, disconnected and inconsistent processes suddenly perform well—in short don’t skip steps ‘A’ and ‘B’ and hope you’ll get the same results by just doing ‘C.’
Too often, operations managers want to skip the basic process improvement steps. Managers may not want to reveal that the status quo of the process state is terribly inefficient, or they may believe that ‘rolling out the robots’ will fix everything.
Examining the operational data can reveal the maturity of an operation. Immature operations regularly have inconsistent handling and routing of work. The good news is that this same data then offers a very clear path to target specific areas of the operation for improvement, prior to a strong push to automate these processes.
2. Use data to rigorously guard against “automation for automation’s sake”
Organizations often have a strong tendency to push for the rollout of automation without considering what the automation will do, or what the core problem is. Automation should be a solution to a defined problem. Automation is definitely not an all-purpose solution to whatever your problem happens to be.
For example, within technology service operations, an automation effort may focus on automating a fix to a recurring issue, such as deleting excess temp files on a disk that keeps filling up, instead of fixing the actual root cause of the problem—e.g., fixing bad code that’s creating that mess in the first place. Automation can certainly help mitigate some short-term symptoms, but it’s not an excuse to avoid old fashioned problem management to fix the root cause behind the symptoms.
I once had an engineer at a meet-up tell me that they felt pressure to avoid traditional problem management because repetitive break-fixes were the easiest to automate—fixing the root causes would lower the number of automated actions in the environment. That, in turn, would lower the automated event numbers reported to senior management for a global automation deployment effort. Don’t be that team.
Would you rather sail on a ‘highly automated’ ship that keeps forming holes in its hull but has a highly trained team of AI robots automatically applying duct tape to each new hole, thus keeping the ship afloat… or would you rather sail on a ship that has far less automation but uses data to manage ship performance and make sure that holes don’t form in the hull in the first place? Most people would choose the latter.
If automation in your organization is taking the form of ‘automated duct tape appliers’ then stop and regroup.
3. Drive to “the money” from the start and make it known
There is a maturity lifecycle that data-driven efforts take within most large corporations. In the early days, money is thrown at hiring talent, building out infrastructure and running new projects. Inevitably, the buzz eventually wears off and people start questioning the value of all these actions. The want to see “the money.”
“The money” in this case can be cost savings, increased revenue, mitigated risks, improved customer satisfaction or some combination thereof—but at some point that should all distill down into improved financial performance for a business.
Above I cited the case of the engineer who was being pressured to increase automation in order to keep the numbers reported to management looking good. This is also a good example of not driving to “the money.” Having more automation in the environment isn’t inherently a good thing nor will it automatically return the value that sponsors want.
The wrong metrics will drive the wrong behaviors. In our infrastructure scenario, someday someone is going to run the numbers and wonder why—despite extensive automation in the environment—the environments are still seen as broadly unstable, the number of P1 incidents hasn’t decreased and costs haven’t gone down. When that mismatch between action, reporting on that action, and “the money” becomes known, it can be a painful and expensive realization for organizations.
Get ahead of that by rigorously tracking “the money” from the beginning. What are you truly trying to accomplish within the operation and is that what you’re actually measuring?
Measurements like the ‘number of automated events’ or ‘% of activities automated’ might make for good headlines, but are they what you are really trying to influence within the business? What if those incidents shouldn’t exist in the first place? Are the right actions being encouraged if automating something that should have been eliminated/avoided is counted as a win?
Nicholas Hartman is the Chief Innovation Officer at CKM Analytix