Too many tools… not enough carpenters!
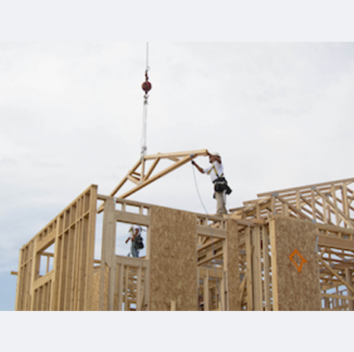
4 min read
Don’t let your enterprise make the expensive mistake of thinking that buying tons of proprietary tools will solve your data analytics challenges.
tl;dr = The enterprise needs to invest in core data science skills, not proprietary tools.
Most of the world’s largest corporations are flush with data, but frequently still struggle to achieve the vast performance increases promised by the hype around so called “big data.” It’s not that the excitement around the potential of harvesting all that data was unwarranted, but rather these companies are finding that translating data into information and ultimately tangible value can be hard… really hard.
In your typical new tech-based startup the entire computing ecosystem was likely built from day one around the need to generate, store, analyze and create value from data. That ecosystem was also likely backed from day one with a team of qualified data scientists. Such ecosystems spawned a wave of new data science technologies that have since been productized into tools for sale. Backed by mind-blowingly large sums of VC cash many of these tools have set their eyes on the large enterprise market. A nice landscape of such tools was recently prepared by Matt Turck of FirstMark Capital (host of Data Driven NYC, one of the best data science meetups around).
Consumers stopped paying money for software a long time ago (they now mostly let the advertisers pay for the product). If you want to make serious money in pure software these days you have to sell to the enterprise. Large corporations still spend billions and billions every year on software and data science is one of the hottest areas in tech right now, so selling software for crunching data should be a no-brainer! Not so fast.
The problem is, the enterprise data environment is often nothing like that found within your typical 3-year-old startup. Data can be strewn across hundreds or thousands of systems that don’t talk to each other. Devices like mainframes are still common. Vast quantities of data are generated and stored within these companies, but until recently nobody ever really envisioned ever accessing — let alone analyzing — these archived records. Often, it’s not initially even clear how the all data generated by these systems directly relates to a large blue chip’s core business operations. It does, but a lack of in-house data scientists means that nobody is entirely even sure what data is really there or how it can be leveraged.
Most large companies have likely also dabbled in ‘data warehousing’ at some stage, but many of these projects have turned into unmanageable blobs of disconnected inconsistent and incomplete datasets. The point being, even if a vendor really could produce a magic black box that could analyze datasets and produce amazing results, there would be nowhere to plug the box in!
Many fail to understand that the core issue is not a lack of tools but a lack of carpenters.
I’ve sat at the table with some of our Fortune 500 clients as one after another hot Silicon Valley startup came in to show off their plug-and-play data tools — only to reveal themselves to be totally clueless with what the data environment inside a large enterprise looks like. The sales teams worked from a slick script working through simple sample datasets, but their consistent inability to answer questions about how the tool would deal with the realities of the enterprise was outright cringe worthy.
In many cases it wasn’t even clear if their product would even solve a real defined business problem. The obvious objective of the sales team was to get the prospective client to just dump all their data into this wonderful new platform (obviously so they could then lock everything into this black box and then start selling more and more licenses). What the tool could actually do (beyond the sales demo) or how it would add value for the business was mostly an afterthought. “Let’s just start loading data and then we’ll figure out how [insert name of tool] can help you!” If you find yourself in a meeting like this, run away as fast as you can!
Needless to say these companies pushing their proprietary toolsets didn’t get the sale. Some of that was just the naivety of young tech startups, but at its core was a fundamental failure to understand that the enterprise’s current difficulty with data analytics isn’t a lack of tools — it’s a lack of carpenters.
More specifically, the enterprise needs:
- Plumbers to make sure all the data flows to the right place where it can be integrated and harvested
- Detectives/Designers with strong data science skills to identify leads in the data and build those out into actionable opportunities for value generation
- Data driven leaders that can take these insights and elicit tangible change in large organizations
- A data centric culture which demands that business operations leverage all available factual information to drive efficiency and effectiveness
Large enterprises move across a data science maturity curve as they seek to derive net new value from their data. Those early in the curve are typically super excited by the prospect of new tools. The promise that “the new system” will produce amazing analytics insights is almost intoxicating. They make a few initial tool purchases, but 6-24 months later end up sorely disappointed that these tools didn’t live up to expectations. The organization then moves to the next level of maturity, realizing that if it wants to fully harvest the power of data it needs to focus on investing in its organization and skills, rather than tools.
The truth is that a top team of data scientists can achieve great results using the toolsets already in place within a large enterprise, supplemented with some free (or very inexpensive) open source tools. This is broadly the approach that our own data science teams take when working with clients.
Proprietary tools won’t replace skilled talent, and skilled talent doesn’t typically need many proprietary tools.
An organization that lacks such data science talent could spend many millions on data analytics tools and still not come anywhere close to the same results. Our own clients are often surprised to learn that our results are typically founded in raw talent combined with tools they already had or simple open source products brought to the table. When someone asks me to show them “the tool” that created these results, I introduce them to our data scientists.
For the large enterprise, the challenge is finding and developing that talent base. For the software companies, the challenge is dealing with the headwinds faced when customers realize they can’t solve their problems by buying more licenses.
Ultimately, this gradual evolution in the market is good for data science as it means more companies are moving further along the maturity curve. It’s probably not so good for those investing in companies that are hoping to sell pre-packaged data analytics tools to the enterprise. There will be exceptions for sure, but the tide is clearly shifting away from the idea that to be more data-driven the enterprise needs to be buying more software licenses. That changing mindset is at least partially responsible for the sales slowdowns and resulting thrashing on the equity markets that many of those selling pre-packaged analytics tools are currently experiencing.
We’re just scratching the surface of what the next wave of innovations in data science can do for large enterprises. Our business is dedicated to helping clients realize that potential. If your business is on that journey by all means admire the shiny tools on display in the conference exhibit hall, but before you cut a purchase order ask yourself: “Do I have highly skilled carpenters that can make something wonderful with this shiny new hammer?” If not, then you’re definitely investing in the wrong place.