From Data-Science Consulting to Real-Time Intelligence Engine
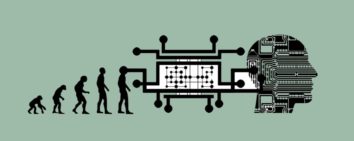
4 min read
In addition to bringing AI and machine learning to the table, data scientists contribute several key elements that elevate consulting engagements: sophisticated real-time analyses, integration of data from multiple sources, ethical algorithms, and insight granularity. When it comes to improving the efficiency of large complex organizations, time is of the essence. Stakeholders are busy, business operations are changing constantly, and new data is being generated all the time. This article explains how data scientists at CKM have evolved our delivery model to bring the most up-to-date, informative, and actionable analysis to our clients.
Our clients typically come to us with very specific questions that can only be answered through intensive data analysis:
- How can we optimize our staffing to match forecasted demand?
- Can we anticipate which employees are likely to resign?
- Where is there wastage/toil in our current processes?
- How do we most efficiently balance contract penalties with excellent customer service and maximized profit margins?
It is impossible to answer these questions without the proper tools and statistical expertise. CKM unifies all possible data sources to create an exhaustive picture of the organization’s business and returns to stakeholders with visualizations and recommendations for how to improve service, maximize efficiency, and minimize risk.
Stone Age Tools: PowerPoint and Other Antiquities
Early on in our presentations (circa 2011-2016), there was PowerPoint. At project finish, we would deliver our final insights using slide decks showing custom-built data visualizations to quantify areas of operational improvement. These were static images developed using the classic data scientist’s toolkit of pandas, NumPy, Matplotlib, Plotly, and seaborn. While we were able to deliver customized analyses developed for a specific client and present them clearly and concisely, there were a couple of obvious drawbacks to this approach.
First, the organizational and technical effort of these projects often led to longer-than-expected durations. To break it down: collecting and validating data (1-4 weeks), developing analysis (1-2 weeks), scheduling presentations, often for multiple stakeholders (1 week), and executing follow-up work based on client feedback (1-2 weeks). For those keeping score, we’re now at 1-2 months. And this does not even begin to cover how long it takes to actually implement the recommended operational changes. From our experience, depending on the amount of bureaucratic red tape, getting these action items signed, sealed, and delivered would take organizations an additional 1-3 months (or more). By this time, the analysis was months out-of-date, and thus the organization was at risk of implementing changes that did not align with the current state of affairs.
Second, the final deliverables were static presentations, meaning that any further questions inspired by the analyses (and there always are further questions) would have to be addressed at a later date with follow-up analyses. Take an example of a project with a global service desk: in one analysis, we identified areas of repetitive work and issues that could be automated away using self-service solutions across the desk. This was a breakdown the client had never seen before, as they did not have any text-mining solutions in their toolkit. They were immediately interested in seeing where these opportunities differed across specific areas of their organization. While we were always happy to provide follow-up work, as it meant our clients were interested in the analysis and recognized its power, we wanted to keep in mind the consequences of the first point mentioned above; the longer it took to settle on an action plan, the more out-of-date the analysis became. So, we began taking steps to fill the gaps.
Building the Tools of the Future: Real-Time Deliverables
To address this first problem of delayed delivery, our team recognized the potential to streamline our analysis by building out a library of pre-packaged scripts. These Python classes span a collection of 20+ analyses that CKM data scientists had identified as beneficial to a wide range of clients, from global IT service desks to large recruiting organizations. These include ticket categorization, capacity vs. demand, productivity, and process mining tools. The automation of these analyses and the common pre-processing steps brought the typical delivery window from 2+ weeks down to just a few days. Additionally, due to the automated nature of these scripts, our data scientists were able to more flexibly and quickly build analysis pipelines that could be updated with new data. All of this work resulted in tightening the gap between the story presented in our final deliverables and the current state of our clients’ organizations so that stakeholders were acting on the most up-to-date information.
In response to the second problem, we pivoted away from slide decks, and in their place developed our own interactive dashboards used as a presentation tool, built using a custom Python, HTML, and CSS library designed in-house. This container holds interactive visuals built in Plotly and D3 that allow our data scientists to develop and present stories we discover within the data quickly and flexibly while also giving our clients the opportunity to really engage with the material. If a stakeholder with specific industry knowledge identifies a specific aspect of the analysis as important, we are able to deep dive into those areas during the presentation. Interactive visuals allowing for impromptu drill-downs and multiple callouts transformed our presentations into guided collaborative workshops, as opposed to the more rigid reports of the past. We have found that this style builds more trust in our analyses and results in more productive conclusions with fewer follow-ups required.
While this delivery model improved the gap between our point-in-time recommendations and the ever-rolling train of business evolution, it is still at its core a temporary fix, a one-time solution. What happens six months after our engagement has ended and the organization is now facing new challenges? How will the organization know that the fixes they implemented the first time around are holding and producing value? One way forward would be to hire our data scientists on an ongoing basis and continuously run these diagnostics month after month. But there is an even more elegant solution.
Personalized Data-Analysis Automation: T-K Blazes a New Path Forward
This journey is what eventually led CKM to develop its own product that automatically addresses all of these problems core to data science consulting and moves our solution into continuous operational improvement – a permanent fix to the problems of data-lag and inflexible insight granularity that also offers an intuitive and exciting user experience. T-K, a CKM product named after the “toolkit” of modules developed over time by our data scientists, connects directly to an organization’s data sources, refreshing analyses in real-time, tracking progress towards goals, automatically providing actionable recommendations, and offering endless options for drilling down into an organization’s complex business operations. It has been a real pleasure to watch our company transition from one-off PowerPoints to building a flexible, real-time product, and we are eager to see how T-K will evolve and bring value to clients across industries. It is now an intelligence engine that allows operational managers to realize initial opportunities and then maintain the highest levels of service, efficiency, and risk mitigation on a continuous basis by adapting to their organization’s changing environment.